Autonomous Truck
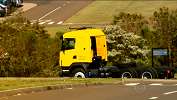
Collaboration between LRM and Scania presents the first fully autonomous truck in Latin America. The prototype is an 9ton. Scania G360 equipped with sensors and an onboard computer allowing safe and efficient navigation with no human intervention.
[More info] [Oficial release] [Photos]
CaRINA 2 - Autonomous Vehicle
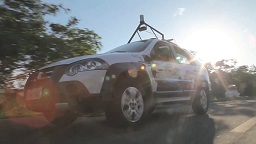
CaRINA 2 is a robotic platform used in the development of perception, control and decision making for autonomous navigation in urban environments. It features computational control of steering and speed, and several on board sensors, such as: GPS, IMU, cameras e laser range finders.
Multi-vehicle Simulation Framework
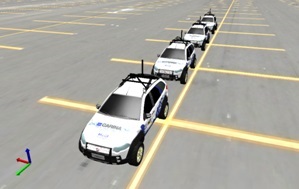
This work proposes a simulation framework for the development of cooperative driving systems. Our framework is capable of accurate simulation of vehicles, sensors, complex environments, and wireless communication between vehicles. It is also compatible to well known robotic control middlewares, allowing portability of the developed code between real and simulated test vehicles.
[Related papers: ITSC2014] [More info] [Videos]
Obstacle and Road Detection
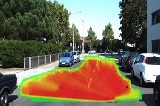
Efficient and robust Obstacle and Road detection are fundamental capabilities to autonomous vehicles. LRM has several ongoing works on this topic based different sensors like: single cameras, stereo cameras and LIDAR. Most approaches have been designed to work on urban environments but we are also interested in agricultural spaces.
[Related Papers: IV2013 IV2014 ITSC2014 ROB2015] [Videos]
Road Detection Using Deep Learning
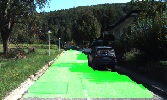
Road detection is a crucial task in autonomous navigation systems. It is responsible for delimiting the road area and hence the free and valid space for maneuvers. In this work, we consider the visual road detection problem where, given an image, the objective is to classify every of its pixels into road or non-road. We address this task by proposing a convolutional neural network architecture. We are especially interested in a model that takes advantage of a large contextual window while maintaining a fast inference. We achieve this by using a Network-in-Network (NiN) architecture and by converting the model into a fully convolutional network after training.
[Related papers: ICRA2016]
Obstacle Detection and Segmentation
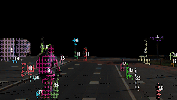
Obstacle Detection is a fundamental problem in autonomous navigation. This work addresses the segmentation of general obstacles using stereo vision with no need of dense disparity maps or assumptions about the scenario. A sparse set of points are selected based on a local spatial condition. These points are then clusterized based on the disparity values and its neighborhood.
[Related papers: IROS2015(W)] [Videos]
Curb and road marking detection for vehicle localization
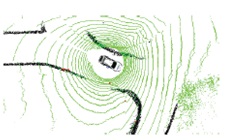
A correct perception of the traffic elements is required for autonomous cars to follow the traffic codes. Curb and road marking are necessary to keep the cars in the streets and in the correct lanes. These features can also be mapped and used vehicle's localization estimation.
[Artigos relacionados: IV2014 ITSC2014 T-ITS2015] [Videos] [More Info] [Download Available]
Autonomous Vehicle Control
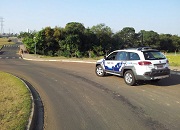
This work proposes a lateral and longitudinal control architecture based on dynamic model inversion for driving near tire-road friction saturation limits for front wheel driven autonomous ground vehicles. Stable cornering conditions near the limit of lateral acceleration are calculated by nonlinear tire models. The control law also takes into account the feasibility of the control path through the phase plane.
[Related papers: IV2014 ITSC2014]
Automatic Mapping of Urban Environments
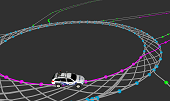
Road network maps are highly used for autonomous vehicle path planning. This work proposes a novel method for automatic construction of road network maps. Collected GPS points are stored into flexible waypoint data structures that can represent relevant information for vehicle navigation. The mapping method also reduces the amount of waypoints by identifying and converting them into lane structures.
[Related papers: LARS2014]
Detection of Eucalyptus Deceases from Aerial Images
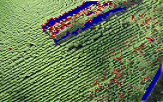
One of the challenges in precision agriculture is the detection of diseased crops in agricultural environments. This work (in cooperation with LSEC Lab) proposes a methodology to detect the diseases in Eucalyptus crops. An unmanned aerial vehicle is used to obtain high-resolution RGB images of a predefined area. The methodology enables the extraction of visual features from image regions and uses several supervised machine learning (ML) techniques to classify regions into three classes: ground, healthy and diseased plants.
[Related papers: ICRA2015]
Path Planning Under Uncertainty for UAVs
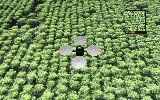
Airborne monitoring in agriculture can a substantially impacts on the identification of diseases and produce accurate information on affected areas. The problem can be formulated as a classification task on aerial images with significant opportunities to impact other fields. This work proposes an active learning method through route planning for improvements in the knowledge on visited areas and minimization uncertainties about the classification of diseases in crops. Binary Logistic Regression and Gaussian Process are used for the detection of pathologies and map interpolation, respectively. A Bayesian optimization strategy is also proposed for the planning of an informative trajectory, which results in a maximized search for affected areas in an initially unknown environment.
[Related papers: ICRA2016]
Tree Counting and Segmentation from Aerial Images
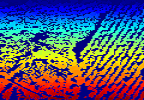
An individual tree crown analysis from aerial images can provide an improvement in economic planning and management of planted forests, as well as identifying diseases on individual trees. To achieve an automated individual tree crown detection, the use of segmentation techniques from image processing becomes a requisite. This work deals on the segmentation and counting of Eucalyptus crops using Near-infrared (NIR) images obtained by UAVs. The proposed methodology employs removing soil using machine learning techniques and then uses segmentation methods from image processing to delineate individual tree crowns.
Past Projects
Obstacle Detection and Classification in Agricultural Environments
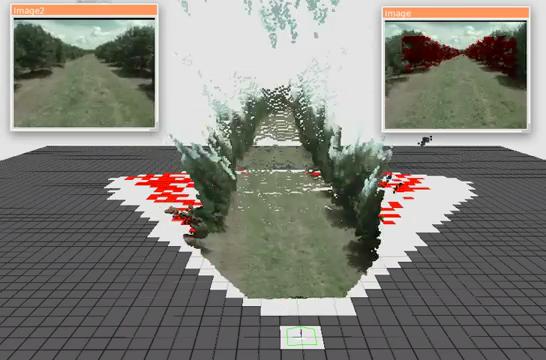
Automation of agriculture machines may reduce human errors, avoid direct human contact to pesticides, and improve efficiency. One of the main challenges of the agricultural machinery automation is a reliable real time obstacle detection system. We propose a robust obstacle detection system that can deal with several types of static and dynamic obstacles and it is also able to distinguish among tall grass, bushes, small animals and people.
[Related papers: ITSC2013]
Road area detection using artificial neural networks
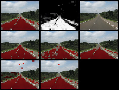
In order to execute autonomous driving in outdoor urban environments it is necessary to identify parts of the terrain that can be traversed and parts that should be avoided. This work describes an analyses of terrain identification based on different visual information using a MLP artificial neural network and combining responses of many classifiers. Experimental tests using a vehicle and a video camera have been conducted in real scenarios to evaluate the proposed approach
[Related papers: JIRS2011 IV2012] [More info] [Download Available]